Note
Click here to download the full example code
Profile the execution of a runtime#
The following example shows how to profile the execution of a model with different runtime.
Training and converting a model#
import numpy
import matplotlib.pyplot as plt
from sklearn.datasets import load_diabetes
from sklearn.ensemble import AdaBoostRegressor
from sklearn.tree import DecisionTreeRegressor
from pyquickhelper.pycode.profiling import profile
from mlprodict.onnx_conv import to_onnx
from mlprodict.onnxrt import OnnxInference
from mlprodict import get_ir_version
data = load_diabetes()
X, y = data.data, data.target
dt = DecisionTreeRegressor()
dt.fit(X, y)
onx = to_onnx(dt, X[:1].astype(numpy.float32), target_opset=11)
oinf = OnnxInference(onx, runtime='python_compiled')
print(oinf)
OnnxInference(...)
def compiled_run(dict_inputs, yield_ops=None, context=None, attributes=None):
if yield_ops is not None:
raise NotImplementedError('yields_ops should be None.')
# inputs
X = dict_inputs['X']
(variable, ) = n0_treeensembleregressor_3(X)
return {
'variable': variable,
}
Profiling and comparison with scikit-learn#
X32 = X.astype(numpy.float32)
def runlocaldt():
for i in range(0, 5000):
oinf.run({'X': X32[:10]})
dt.predict(X[:10])
print("profiling...")
txt = profile(runlocaldt, pyinst_format='text')
print(txt[1])
profiling...
_ ._ __/__ _ _ _ _ _/_ Recorded: 03:21:28 AM Samples: 2763
/_//_/// /_\ / //_// / //_'/ // Duration: 2.777 CPU time: 2.773
/ _/ v4.4.0
Program: somewhere/workspace/mlprodict/mlprodict_UT_39_std/_doc/examples/plot_profile.py
2.776 profile ../pycode/profiling.py:455
`- 2.776 runlocaldt plot_profile.py:44
[222 frames hidden] plot_profile, sklearn, <__array_funct...
Profiling for AdaBoostRegressor#
The next example shows how long the python runtime spends in each operator.
ada = AdaBoostRegressor()
ada.fit(X, y)
onx = to_onnx(ada, X[:1].astype(numpy.float32), target_opset=11)
oinf = OnnxInference(onx, runtime='python_compiled')
print(oinf)
OnnxInference(...)
def compiled_run(dict_inputs, yield_ops=None, context=None, attributes=None):
if yield_ops is not None:
raise NotImplementedError('yields_ops should be None.')
# init: axis_name (axis_name)
# init: estimators_weights (estimators_weights)
# init: half_scalar (half_scalar)
# init: k_value (k_value)
# init: last_index (last_index)
# init: negate (negate)
# init: shape_tensor (shape_tensor)
# inputs
X = dict_inputs['X']
(est_label_9, ) = n0_treeensembleregressor_3(X)
(est_label_10, ) = n1_treeensembleregressor_3(X)
(est_label_15, ) = n2_treeensembleregressor_3(X)
(est_label_4, ) = n3_treeensembleregressor_3(X)
(est_label_19, ) = n4_treeensembleregressor_3(X)
(est_label_20, ) = n5_treeensembleregressor_3(X)
(est_label_23, ) = n6_treeensembleregressor_3(X)
(est_label_22, ) = n7_treeensembleregressor_3(X)
(est_label_14, ) = n8_treeensembleregressor_3(X)
(est_label_12, ) = n9_treeensembleregressor_3(X)
(est_label_13, ) = n10_treeensembleregressor_3(X)
(est_label_17, ) = n11_treeensembleregressor_3(X)
(est_label_16, ) = n12_treeensembleregressor_3(X)
(est_label_11, ) = n13_treeensembleregressor_3(X)
(est_label_8, ) = n14_treeensembleregressor_3(X)
(est_label_21, ) = n15_treeensembleregressor_3(X)
(est_label_6, ) = n16_treeensembleregressor_3(X)
(est_label_5, ) = n17_treeensembleregressor_3(X)
(est_label_0, ) = n18_treeensembleregressor_3(X)
(est_label_1, ) = n19_treeensembleregressor_3(X)
(est_label_3, ) = n20_treeensembleregressor_3(X)
(est_label_2, ) = n21_treeensembleregressor_3(X)
(est_label_18, ) = n22_treeensembleregressor_3(X)
(est_label_7, ) = n23_treeensembleregressor_3(X)
(est_label_35, ) = n24_treeensembleregressor_3(X)
(est_label_27, ) = n25_treeensembleregressor_3(X)
(est_label_45, ) = n26_treeensembleregressor_3(X)
(est_label_32, ) = n27_treeensembleregressor_3(X)
(est_label_48, ) = n28_treeensembleregressor_3(X)
(est_label_43, ) = n29_treeensembleregressor_3(X)
(est_label_34, ) = n30_treeensembleregressor_3(X)
(est_label_29, ) = n31_treeensembleregressor_3(X)
(est_label_40, ) = n32_treeensembleregressor_3(X)
(est_label_39, ) = n33_treeensembleregressor_3(X)
(est_label_38, ) = n34_treeensembleregressor_3(X)
(est_label_46, ) = n35_treeensembleregressor_3(X)
(est_label_33, ) = n36_treeensembleregressor_3(X)
(est_label_26, ) = n37_treeensembleregressor_3(X)
(est_label_42, ) = n38_treeensembleregressor_3(X)
(est_label_25, ) = n39_treeensembleregressor_3(X)
(est_label_30, ) = n40_treeensembleregressor_3(X)
(est_label_49, ) = n41_treeensembleregressor_3(X)
(est_label_36, ) = n42_treeensembleregressor_3(X)
(est_label_28, ) = n43_treeensembleregressor_3(X)
(est_label_44, ) = n44_treeensembleregressor_3(X)
(est_label_41, ) = n45_treeensembleregressor_3(X)
(est_label_37, ) = n46_treeensembleregressor_3(X)
(est_label_24, ) = n47_treeensembleregressor_3(X)
(est_label_31, ) = n48_treeensembleregressor_3(X)
(est_label_47, ) = n49_treeensembleregressor_3(X)
(concatenated_labels, ) = n50_concat(est_label_0, est_label_1, est_label_2, est_label_3, est_label_4, est_label_5, est_label_6, est_label_7, est_label_8, est_label_9, est_label_10, est_label_11, est_label_12, est_label_13, est_label_14, est_label_15, est_label_16, est_label_17, est_label_18, est_label_19, est_label_20, est_label_21, est_label_22, est_label_23, est_label_24, est_label_25, est_label_26, est_label_27, est_label_28, est_label_29, est_label_30, est_label_31, est_label_32, est_label_33, est_label_34, est_label_35, est_label_36, est_label_37, est_label_38, est_label_39, est_label_40, est_label_41, est_label_42, est_label_43, est_label_44, est_label_45, est_label_46, est_label_47, est_label_48, est_label_49)
(negated_labels, ) = n51_mul(concatenated_labels, negate)
(sorted_values, sorted_indices, ) = n52_topk_11(negated_labels, k_value)
(array_feat_extractor_output, ) = n53_arrayfeatureextractor(estimators_weights, sorted_indices)
(reshaped_weights, ) = n54_reshape_5(array_feat_extractor_output, shape_tensor)
(weights_cdf, ) = n55_cumsum(reshaped_weights, axis_name)
(median_value, ) = n56_arrayfeatureextractor(weights_cdf, last_index)
(comp_value, ) = n57_mul(median_value, half_scalar)
(median_or_above, ) = n58_less(weights_cdf, comp_value)
(cast_result, ) = n59_cast(median_or_above)
(median_idx, ) = n60_argmin_12(cast_result)
(median_estimators, ) = n61_gatherelements(sorted_indices, median_idx)
(variable, ) = n62_gatherelements(concatenated_labels, median_estimators)
return {
'variable': variable,
}
The profiling.
def runlocal():
for i in range(0, 500):
oinf.run({'X': X32})
print("profiling...")
txt = profile(runlocal, pyinst_format='text')
print(txt[1])
profiling...
_ ._ __/__ _ _ _ _ _/_ Recorded: 03:21:33 AM Samples: 9733
/_//_/// /_\ / //_// / //_'/ // Duration: 246.075 CPU time: 1345.086
/ _/ v4.4.0
Program: somewhere/workspace/mlprodict/mlprodict_UT_39_std/_doc/examples/plot_profile.py
246.075 profile ../pycode/profiling.py:455
`- 246.074 runlocal plot_profile.py:72
[179 frames hidden] plot_profile, mlprodict, <string>, <b...
240.662 TreeEnsembleRegressor_3._run mlprodict/onnxrt/ops_cpu/op_tree_ensemble_regressor.py:102
With a different runtime#
Let’s compare to onnxruntime.
onx.ir_version = get_ir_version(11)
oinf = OnnxInference(onx, runtime='onnxruntime1')
def runlocalort():
for i in range(0, 500):
oinf.run({'X': X32})
print("profiling with onnxruntime...")
txt = profile(runlocalort, pyinst_format='text')
print(txt[1])
profiling with onnxruntime...
_ ._ __/__ _ _ _ _ _/_ Recorded: 03:25:43 AM Samples: 500
/_//_/// /_\ / //_// / //_'/ // Duration: 1.448 CPU time: 5.770
/ _/ v4.4.0
Program: somewhere/workspace/mlprodict/mlprodict_UT_39_std/_doc/examples/plot_profile.py
1.447 profile ../pycode/profiling.py:455
`- 1.447 runlocalort plot_profile.py:91
[5 frames hidden] plot_profile, mlprodict
py-spy#
py-spy may be used to dig into native functions. An example can be found at: Profiling AdaBoostRegressor. The last piece of code uses the standard python profiler.
pr, df = profile(runlocal, as_df=True)
ax = df[['namefct', 'cum_tall']].head(n=15).set_index(
'namefct').plot(kind='bar', figsize=(8, 3), rot=15)
ax.set_title("Simple profiling")
for la in ax.get_xticklabels():
la.set_horizontalalignment('right')
plt.show()
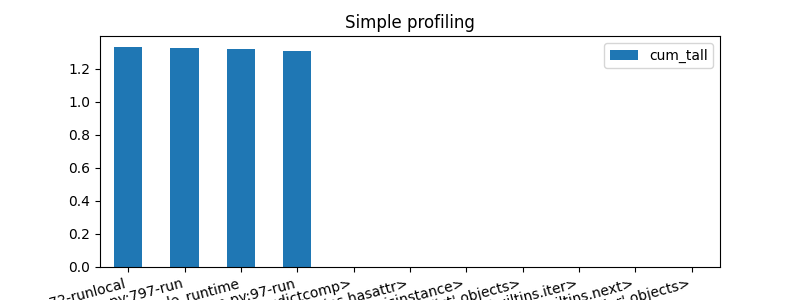
somewhere/workspace/mlprodict/mlprodict_UT_39_std/_venv/lib/python3.9/site-packages/pyquickhelper/pycode/profiling.py:541: FutureWarning: The default value of numeric_only in DataFrameGroupBy.sum is deprecated. In a future version, numeric_only will default to False. Either specify numeric_only or select only columns which should be valid for the function.
df = df.groupby(['namefct', 'file'], as_index=False).sum().sort_values(
Total running time of the script: ( 4 minutes 19.026 seconds)